For research use only. Not for use in diagnostic procedures.
Answer
1) Baseline and Advanced Baseline Correction
Advanced baseline correction is useful in processing noisy linear mode (high mass) spectra (e.g., Protein or DNA samples).
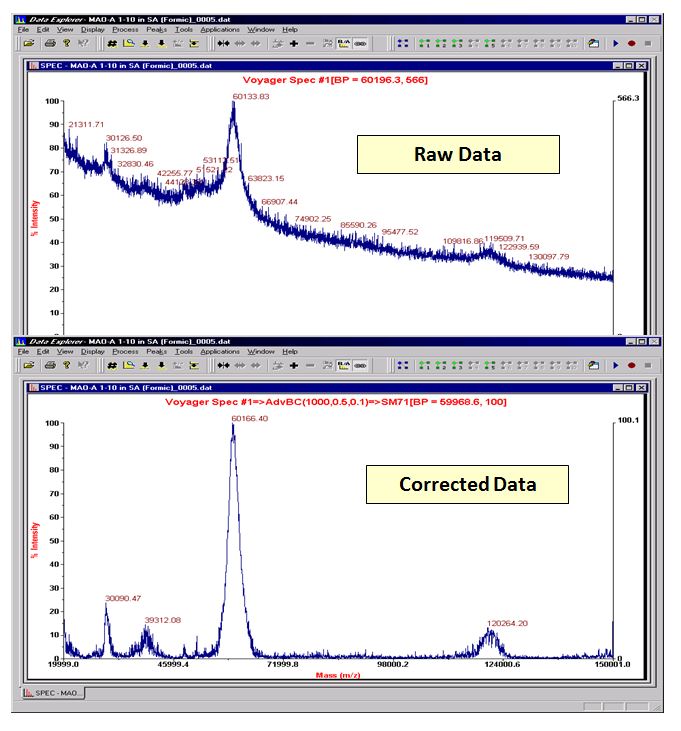
2) Types of Noise Reduction
- Noise Removal (true denoising)
- Noise Filtering (another true denoising)
- Default Smoothing (resolution based)
- Gaussian Smoothing (classical smoothing)
3) Noise Removal NR (2.00)
This program assumes that noise in the spectrum is white noise. The program reduces random fluctuations that are within the noise range. The noise range is set by the “Std Dev to remove” number that the program requires. Typically this is set at 2, such that any signal below a 2:1 signal-to-noise ratio (RMS noise definition) is removed. This setting provides very aggressive denoising. A setting of 1 is less aggressive. The technique unlike classical smoothing does not reduce the resolution of the peaks to any significant degree.
- Provides very aggressive denoising
- Usually parameter-less, but may want to adjust from 2 to 1 to avoid spikes.
- Retains as close as possible original peak resolution
- Excellent for presentation purposes
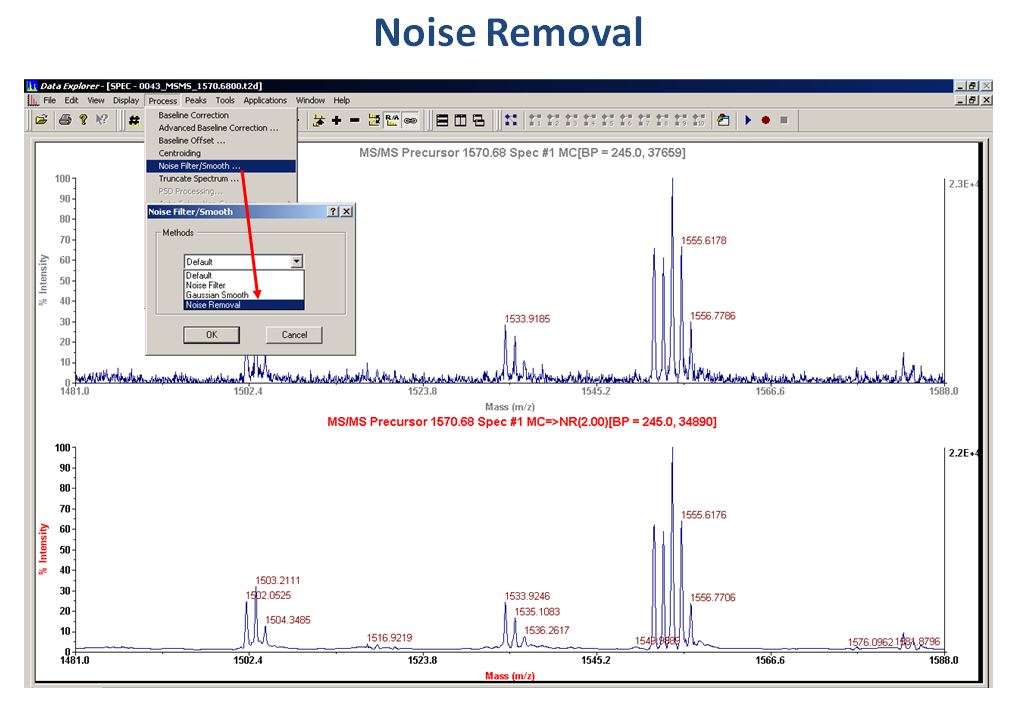
4) Noise Filtering NF (0.7)
Unfiltered noise is random and uncorrelated, while signals are highly correlated, in that successive y-values progressively reduce from peak tops. The noise-filtering program is designed to identify correlated and uncorrelated features in data so that only the uncorrelated noise components are filtered. The degree of correlation is the only parameter and determines how efficiently the noise is filtered with respect to the signals. This value typical varies from 0 to 1 with a common default of 0.7. Minimum resolution loss across peaks is typically observed.
- Virtually parameter-less, and the default of 0.7 is best for most data.
- Allows resolution to be maintained while reducing noise.
- Retains small peak features near the baseline
5) Default smoothing (resolution-based gaussian)
This function is an advance on classical gaussian smoothing. The filter is changed across the mass range depending upon the resolution setting stated in the peak detection parameters. Classical smoothing will tend to over smooth at the low mass end and under smooth at the high mass end. The program is parameter-less, but is dependent on the setting in the peak detection parameters.
6) Smoothing
This the classical smoothing program which allows user interaction via setting the number of smoothing points (from 3 up to 353). The program defaults to a “suggested” value for the acquired trace (back-highlighted in blue) for ease of use.
- Is a known quantity.
- Allows user input over a wide range
- Data output can be compared with other data outputs
Posted: now